A Simulation-based Decision Guide to Navigate Missing Data in Clinical Studies
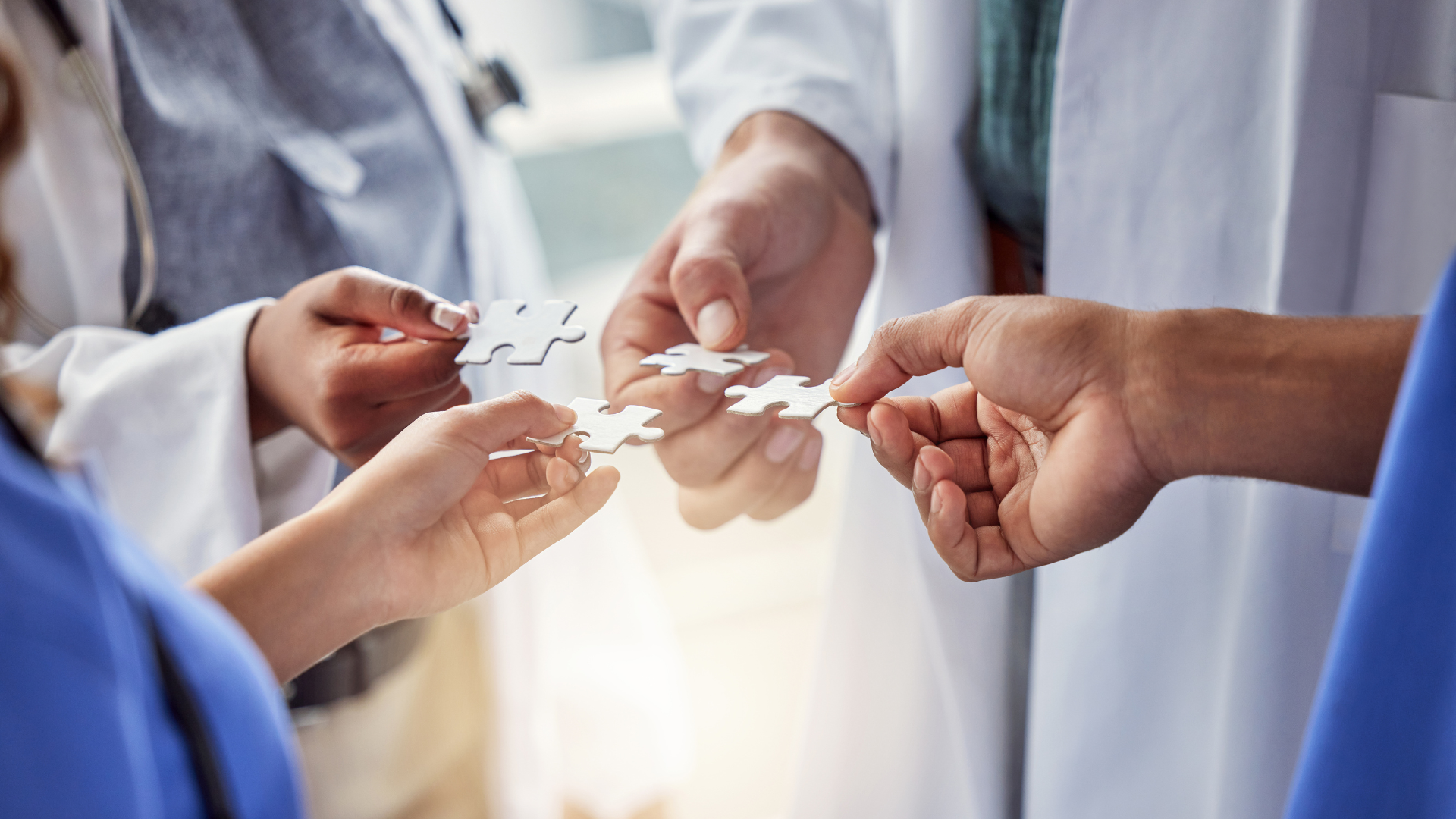
Missing Data – A Common But Critical Issue in Clinical Studies
In part one, of this two part blog series, we will discuss the missing data issue in clinical studies. In bioavailability (BA) and bioequivalence (BE) studies with pharmacokinetic endpoints, the occurrence of missing data points, is an inevitable challenge faced in clinical studies. Several factors can lead to this issue, including but not limited to dropouts, non-compliance, unanticipated complications in sample access or handling, and bioanalysis. These can undermine the precise determination of pharmacokinetic parameters, diminish statistical power, and impede solid bioavailability and bioequivalence conclusions.
While efforts are employed during study design or execution to mitigate such effects through strategic sampling time and rigorous training to ensure protocol adherence, the occurrence of missing data points remains intrinsic to clinical studies. Acknowledged by regulatory agencies like the ICH and EMA, there is no universally accepted gold standard to account for missing data in pharmacokinetic and statistical analysis, or for defining acceptable levels of maximum missing observations. In particular, EMA indicates that if missing observations were to be handled by excluding the subject from the analysis, this could weaken statistical power.
Furthermore, non-completers might also possess extreme values that could skew the variability estimate and bias treatment effects. Consequently, post-hoc sensitivity analysis is recommended in cases of substantial unexpected missing data to justify and reinforce treatment effect conclusions.
Methods to Address Missing Data:
There are numerous methods for addressing missing data in bioavailability and bioequivalence studies, but this blog post explores the discussion, focusing on four common approaches.
- Complete Case Analysis method, or listwise deletion, involves including solely on subjects with complete data while omitting those with any missing values from the statistical analysis. Although it is a reasonable strategy with adequate sample size and study power, it could introduce bias into pharmacokinetic parameter estimations and undermine the real treatment effect due to impactful missing data.
- Pairwise Deletion eliminates specific missing data point(s) while retaining all other data for analysis. Though it preserves more data than listwise deletion, it faces limitations when a high number of missing observations is present.
- Statistical MIXED Model utilizes a method called Restricted Maximum Likelihood to estimate parameters using all available data. This method’s advantage lies in its ability to provide unbiased estimates by leveraging the conditional distribution of other variables, even with unbalanced data.
- Sensitivity Analysis evaluates study robustness under various missing data scenarios, which provides insights into the potential impact of missing data on study findings.
Published Insights from Simulated Missing Time Points in Bioavailability and Bioequivalence Studies
Although analysis methods described above and other pharmacokinetic modelling on how to handle missing data are extensively studied, limited research has investigated the effect of missing data on both bioavailability and bioequivalence conclusions and the estimation of pharmacokinetic parameters.
Only one article in the public domains discussing the potential impact of bioequivalence due to missing pharmacokinetic data point was found at the time of this post. Donner and colleagues conducted over 10,000 simulations to evaluate the impact of missing time points in bioequivalence studies.
Their simulation studies encompassed varying subject counts of 15, 20, or 25 subjects, featuring four missing patterns: random, early, central, or late, and consisting of 12 sampling time points. The simulation explored different scenarios where 1 to 3 subjects had missing data, and each of these subjects had anywhere from 3 to 9 missing time points. The investigators reported several key observations.
- Firstly, the impact of random missing data emerged as relatively minor, remaining inconsequential even when 3 subjects presented up to 7 missing time points each.
- Secondly, the omission of data points corresponding to the terminal concentration-time profile (i.e., the late phase) displayed the most profound effect. Even with as few as four missing time points for a single subject, this led to distorted estimation of the area under the curve (AUC) near the terminal phase.
- Thirdly, an incomplete profile featuring an early, or central missing time point exerted a moderate impact, unless the count of missing time points for an individual subject exceeded 7.
It was concluded that missing data introduces bias, increases variability, and compromises the reliability of study results as only a small proportion of 32 out of 252 simulated and reported scenarios met the established criteria, underlying the susceptibility of result distortion.
Bridging Gaps: Understanding the Impact of Incomplete Pharmacokinetic Data
While the published simulation by Donner and colleague stands as pioneering work in exploring missing time points in bioequivalence studies, further extensive simulations evaluating diverse scenarios could unveil a deeper extent and potential consequences of incomplete pharmacokinetic data on bioavailability and bioequivalence.
The most common approach to handle missing data that affects pharmacokinetic parameter estimation in bioavailability and bioequivalence studies is to exclude the impacted parameter(s) from bioavailability and bioequivalence assessment (akin to pairwise deletion). However, some argue that in certain cases, including affected data could yield advantages for bioavailability and bioequivalence assessment.
The criteria used to determine the severity of the impact on pharmacokinetic parameter is also controversial. Therefore, we will conduct simulations with various degree of incomplete sampling and investigate the impact of including or excluding affected parameters on bioavailability bioequivalence studies outcomes.
Concluding Remarks for Future Simulations
Despite diligent study design and diverse analysis strategies are utilized to minimize the impact of missing data, the presence of missing observation remains an inherent facet in bioavailability and bioequivalence studies. Particularly, the implications of incomplete pharmacokinetic data remain uncertain. We invite you to stay tuned for our forthcoming simulations, which endeavour to simulate multiple scenarios with missing data and unfold the extent to which missing data can influence bioavailability and bioequivalence studies outcomes.
The goal is to shed light on whether the approach of including or excluding affected subjects should be adopted when providing pharmacokinetic impact assessment. Stay connected as we eagerly look forward to sharing our discoveries and insights in our future blog posts.
Written By: Jacinda Kwok, Pharmacokinetic Scientist